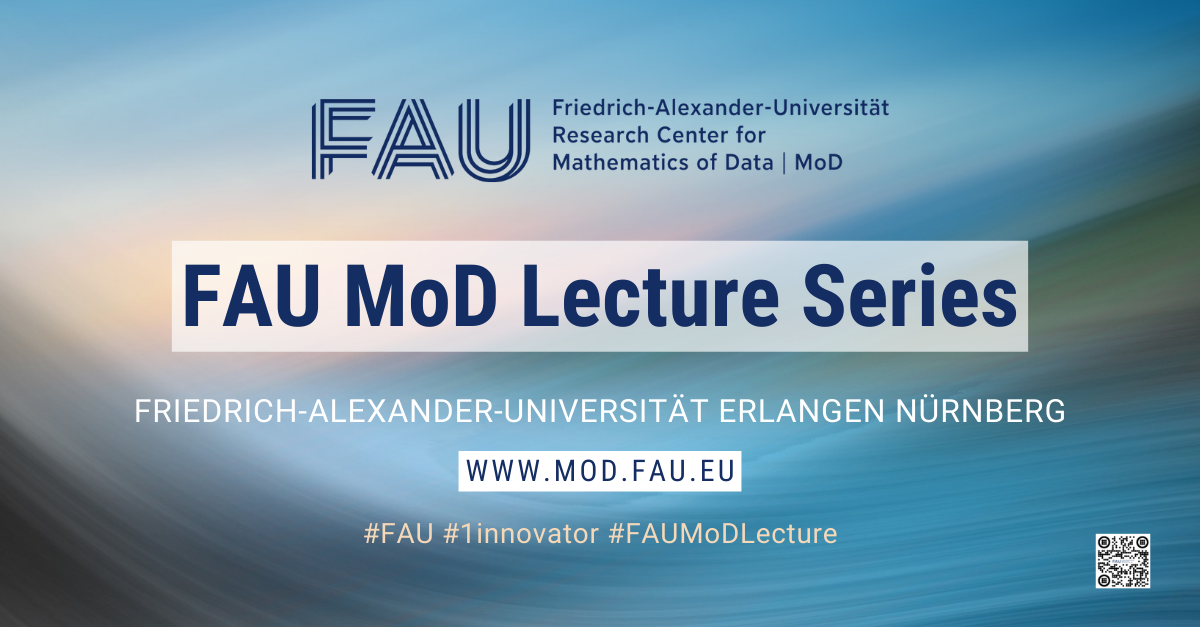
FAU MoD Lecture Series. Special December 2024
Date: Tue. December 03, 2024
Event: FAU MoD Lecture Series (Special double session. December 2024)
Organized by: FAU MoD, the Research Center for Mathematics of Data at Friedrich-Alexander-Universität Erlangen-Nürnberg (Germany)
Session 01: 14:30H
FAU MoD Lecture: The implicit bias phenomenon in deep learning
Speaker: Prof. Dr. Holger Rauhut
Affiliation: Mathematisches Institut der Universität München (Germany)
Abstract. Deep neural networks are usually trained by minimizing a non-convex loss functional via (stochastic) gradient descent methods. Unfortunately, the convergence properties are not very well-understood. Moreover, a puzzling empirical observation is that learning neural networks with a number of parameters exceeding the number of training examples often leads to zero loss, i.e., the network exactly interpolates the data. Nevertheless, it generalizes very well to unseen data, which is in stark contrast to intuition from classical statistics which would predict a scenario of overfitting.
A current working hypothesis is that the chosen optimization algorithm has a significant influence on the selection of the learned network. In fact, in this overparameterized context there are many global minimizers so that the optimization method induces an implicit bias on the computed solution. It seems that gradient descent methods and their stochastic variants favor networks of low complexity (in a suitable sense to be understood), and, hence, appear to be very well suited for large classes of real data.
Initial attempts in understanding the implicit bias phenomen considers the simplified setting of linear networks, i.e., (deep) factorizations of matrices. This has revealed a surprising relation to the field of low rank matrix recovery (a variant of compressive sensing) in the sense that gradient descent favors low rank matrices in certain situations. Moreover, restricting further to diagonal matrices, or equivalently factorizing the entries of a vector to be recovered, shows connection to compressive sensing and l1-minimization.
Session 02: 16:00H
FAU MoD Lecture: Counterintuitive approximations
Speaker: Prof. Dr. Christian Bär
Affiliation: Institut für Mathematik. Universität Potsdam (Germany)
Abstract. The Nash-Kuiper embedding theorem is a prototypical example of a counterintuitive approximation result: any short (but highly non-isometric) embedding of a Riemannian manifold into Euclidean space can be approximated by isometric C¹-embeddings. As a consequence, any surface can be isometrically C¹-embedded into an arbitrarily small ball in ℝ³. For C²-embeddings this is impossible due to curvature restrictions.
I will present a general result which allows for approximations by functions satisfying strongly overdetermined equations on open dense subsets. This will be illustrated by three examples: Lipschitz functions with surprising derivative, surfaces in 3-space with unexpected curvature properties, and a similar statement for abstract Riemannian metrics on manifolds. Our method is based on “cut-off homotopy”, a concept introduced by Gromov in 1986.
This is based on joint work with Bernhard Hanke.
AUDIENCE
This is a hybrid event (On-site/online) open to: Public, Students, Postdocs, Professors, Faculty, Alumni and the scientific community all around the world.
WHEN
Tue. December 03, 2024 at 14:30H (Berlin time)
WHERE
On-site / Online
[On-site] Friedrich-Alexander-Universität Erlangen-NürnbergFelix Klein building. Department Mathematik
Room H13 Johann-Radon-Hörsaal
Cauerstraße 11, 91058 Erlangen
GPS-Koord. Raum: 49.579737N, 11.029862E [Online] FAU Zoom link
Meeting ID: TBA | PIN code: TBA
You might like:
• FAU MoD Lectures
• FAU MoD Lecture: Measuring productivity and fixedness in lexico-syntactic constructions by Prof. Dr. Stephanie Evert
• FAU MoD Lecture: New avenues for the interaction of computational mechanics and machine learning by Prof. Dr. Paolo Zunino
• FAU MoD Lecture: Discovering and Communicating Excellence by Prof. Dr. Ute Klammer
• FAU MoD Lecture: Thoughts on Machine Learning by Prof. Dr. Rupert Klein
• FAU MoD Lecture: Using system knowledge for improved sample efficiency in data-driven modeling and control of complex technical systems by Prof. Dr. Sebastian Peitz
• FAU MoD Lecture: Image Reconstruction – The Dialectic of Modelling and Learning by Prof. Dr. Martin Burger
• FAU MoD Lecture: The role of Artificial Intelligence in the future of mathematics by Prof. Dr. Amaury Hayat
• FAU MoD Lecture: FAU MoD Lecture. Special November 2023 by Prof. Dr. Michael Kohlhase and Prof. Dr. Edriss S. Titi
• FAU MoD Lecture: Free boundary regularity for the obstacle problem by Prof. Dr. Alessio Figalli
• FAU MoD Lecture: Physics-Based and Data-Driven-Based Algorithms for the Simulation of the Heart Function by Prof. Dr. Alfio Quarteroni
• FAU MoD Lecture: From Physics-Informed Machine Learning to Physics-Informed Machine Intelligence: Quo Vadimus? by Prof. Dr. George Karniadakis
• FAU MoD Lecture: From Alan Turing to contact geometry: Towards a “Fluid computer” by Prof. Dr. Eva Miranda
• FAU MoD Lecture: Applications of AAA Rational Approximation by Prof. Dr. Nick Trefethen
• FAU MoD Lecture: Learning-Based Optimization and PDE Control in User-Assignable Finite Time by Prof. Dr. Miroslav Krstic
_
Don’t miss out our last news and connect with us!
www.mod.fau.eu/events